What is Big Data?
Big data is data that is very large or very unstructured. It is the most complex data to analyze. For that you need advanced big data technology and Big Data solutions (tools) that can work with enormous amounts of unstructured data.
Why Big Data analytics? The answer is simple: often there is a wealth of information hidden in big data that can help your business or institution perform much better. You suddenly start to see patterns that you would not have discovered with normal data analysis. You conduct big data research and stumble upon new knowledge that can give you a competitive advantage and/or substantially increase the quality of your services.
Relevant questions that make or break Big Data applications
Every day we are inundated with this enormous amount of data. Somehow you sense that your organization can and must do something with this. After all, the competition is not standing still, technology is developing rapidly, and the market is constantly changing. A number of concrete questions arise:
- What can and should my organization do with Big Data-Analytics?
- What does a successful project look like? When and how do you involve stakeholders in a project?
- What are the risks, pitfalls, and pros and cons of Big Data Science?
- What Big Data applications can I identify in my organization and what is the impact?
- What new business models are enabling big data analytics?
- Which Big Data examples capture the imagination and what can you learn from them?
- Where and how do I store big data? When do you need a data lake?
- What Big Data & analytics tools are available?
- What skills do my people need to make big data management a success?
- How should my organization deal with any big data privacy issues?
- What relevant laws and regulations do you need to consider?
Big Data is volatile, complex, voluminous, and unstructured
Big data can hold unprecedented value for any organization. But the data is also difficult to analyze and apply. Why? Because big data is volatile, complex, large in scope, and unstructured. Think, for example, of satellite images, log files of systems, or sound clips that you can analyze to extract information. The Big Data & analytics specialists of Passionned Group can assist you in obtaining clear insights and clear big data analysis. We are 100% independent and not bound to any supplier. And of course, we are happy to take care of a successful implementation for your organization. Contact us now to get more information.
What is Big Data analytics or Big Data management?
We have now answered the questions “What is Big Data?” and “Why big data?” but have not yet addressed the field.
Big Data Analytics is the field that stores, processes, models, and analyzes big data in an efficient manner. It aims to improve, restructure, and optimize business processes and innovation in organizations.
So how do you go about giving big data meaning in your organization?
- First, by gaining a lot of knowledge about the field. You can do this, for example, by taking a course in Big Data Analytics. You will also take a close look at your primary and secondary business processes and look for big data applications that can have a major impact. You will discuss these with each other and eventually draw up one or more business cases. After all, you want to have an idea in advance of whether it will generate money.
- Finally, you are going to implement big data analytics. Once people see that it works and see the benefits, big data becomes meaningful to them and they can explain big data to others.
Immerse yourself in the field of Big Data Analytics
The field of Big Data Management is particularly interesting because you can start creating predictive models, renew your business model (from reactive to proactive), and implement disruptive innovations. Click on any of the big data articles below to learn more:
- The 7 most important BI & big data trends in 2024
- The 7 biggest big data pitfalls
- The ethics of big data: temptation and fear
- 6 reasons not to build a data lake
Big data examples & applications
In order to learn from other organizations and as inspiration, we provide here a number of appealing examples of big data applications in a number of sectors. What is striking is that the number of examples of big data applications in the public sector is large. There is a logical explanation for this: the public space itself is huge, roughly everything between your home, office, and other destinations.
In addition, photos and video images are easy to take these days, even automatically by having drones fly around with an (infrared) camera. Think, for example, of photos that can indicate whether trees are sick, gardens are tidy, and whether weeds are too high. But the photos also show whether parking spaces are occupied by cars without a valid permit or they indicate the state of maintenance of objects in the outdoor area. There are also numerous examples of big data healthcare. Within healthcare, it is increasingly common to use big data analytics to enable specialists to detect diseases at an early stage, for example.
1. Predicting fires with big data technology
The Amsterdam Fire Department was the big winner of the Dutch BI & Data Science Award. Not only did they get the audience award, but they also took home the prize from the professional jury. The fire department is now able to predict fires even before they break out, based on dozens of (open and large) datasets. Armed with this new knowledge and insight, firefighters now go door-to-door to provide information using an information video shown on a tablet. They show residents how they can prevent certain types of fires themselves, for example by cooking differently and more safely.
2. Optimizing traffic flows with big data & analytics
The Dublin City Council conducted research into the potential of big data analytics. It investigated whether it would be possible to optimize traffic flows in the city and reduce congestion.
Numerous sensors were built into the road surface, as well as GPS systems in the buses. Rain detectors were also installed at key points in the city. Finally, the city government tapped data from all the cameras.
Now that the system is live, in Dublin, they collect all the data in real-time and store it in a data lake. They present the data on a map of the city. This allows them to immediately visualize where traffic jams are likely to occur. Using the camera images, the staff at the traffic control center can immediately see the cause of a traffic jam. And they immediately take the appropriate action.
If there is a serious accident, they immediately alert the police and call an ambulance. Even before those involved in the incident can do so. In other cases, they send traffic controllers to the scene to direct traffic. With this application of Big Data Analytics, they also calculate the most optimal routes for buses through the city.
Would you like to learn more about Big Data tools? Choose one of the tools from the list below:
We have assessed all Big Data tools (and BI tools) in our BI & Analytics Guide 2022 . There you will find all the details of all our solutions.
3. Big data care example: the smart inhaler
Asthma is a common chronic lung disease. The disease affects as many as 339 million people worldwide. It causes premature death and reduces the quality of life in people of all age groups. The smart inhaler helps asthmatics around the world to better control the disease. This Big Data and IoT application collects all kinds of data. For example, it not only measures how a patient’s intake of the medicine is progressing, but it also measures location, air quality, outdoor temperature, humidity, and whether there is pollen in the air. The smart inhaler sends this big data to a data center where a machine learning model determines what action to take.
The machine learning model may conclude that based on the air quality, the patient’s previous seizures, and the location, that the patient would be wise to inhale the medicine within five minutes at a dosage determined by the algorithm. Or the algorithm alerts the departing patient on his or her smartphone that the inhaler is still at home. Or the algorithm sends the patient a notification that he or she is driving into an area where a lot of grass pollen is active in the air. The smart inhaler thus helps asthmatics to reduce the number of attacks and helps them to find out what caused attacks. A great example where AI and Big Data Science are intertwined in a product with great social impact.
4. Predictive Maintenance & Big Data
This Big Data application is often used to predict defects in machines. Sensors are placed on or in certain parts of the machine that measure, for example, the temperature. Based on patterns visible in the data, an algorithm can predict with a fairly high probability that a part or parts are likely to break down soon. Predictive Maintenance with Big Data can prevent high failure rates and thus save a lot of money. In addition, it also has a major impact on customer satisfaction.
Why every controller should know everything about Big Data
Think of a useful Big Data application first
What makes the Dublin case very clear is that they came up with a relevant application beforehand. This is the most crucial step before you get started with Big Data management and rig a mature architecture. What better or faster decisions can you make based on that data? Too often, the focus in this field is on data storage or Big Data tools. And not on what it can yield and what new business models it makes possible. The result is that the data is not profitable and the big data “machine” quickly crashes.
The Data Science book for Decision Makers & Data Professionals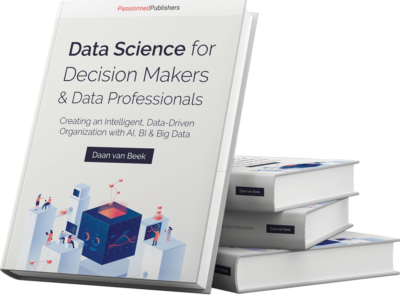
Principles and characteristics of Big Data: the five Vs
Big data is characterized by a number of features, we call them the 5 V’s. One or more of the following situations can be considered Big Data:
- Volume: how big is Big Data? The data volume is so large that the data no longer fits into a traditional SQL database. Storage takes place in file systems or so-called NoSQL databases. Extracts are stored in the data warehouse.
- Velocity: the data appears quickly and can disappear again very quickly. Twitter, for example, moves older tweets to an archive. That data evaporates quickly. Machine data (IoT Big Data) even evaporates almost immediately. So, you have to be there very quickly to catch the data.
- Variety: the data has a lot of variation in structure, volume, and meaning.
- Veracity: varying data quality and doubts about the reliability of the data make the use of big data questionable.
- Value: this is what really matters, what value will big data bring to your customers and your organization?
Figure 1: To understand the true value of Big Data, you first need to understand the five well-known V’s that characterize Big Data
You can clarify the principles of Big Data with the characteristics of Big Data, but this does not tell the whole story. Especially when it comes to image processing. Because of this specific application, we also call photography the new universal language, because based on photos you can, with great precision and speed, relatively easily identify defects in your products, but also detect incipient diseases in a human, animal, or plant. The application possibilities of image processing are enormous, especially in combination with robots, Artificial Intelligence, and drones.
Types of Big Data and open data
Whether it is Big Data, normal data, or open data, the storage takes place on computers that work with bits (zeros and ones). At this level, you can’t see any difference between these types of data. But at a higher level, you can discover the following types of big data:
Documents: think of emails, quotes, policy memos and text files
- Photos: taken with your phone, a camera, or special (hospital) equipment
- Videos: can be taken with your phone, a video camera, or more advanced equipment
- Sound fragments: these are recorded with a sound recorder
- Sensory or machine data: these are generated by machines
- RFID tags: think of wristbands or stickers with a chip that you can detect
- Social media messages: these are created by the user
- Log files: this Big Data is generated by computers, websites, and systems (event logs)
Maybe you’re not taking pictures or recordings right now, or you don’t know about the log files that all the computers or routers in your company are already generating. In order to achieve success with Big Data, it is necessary to go through all types of Big Data in a structured way. Check whether in your organization you can easily identify this data and see who or what generates what data.
Big data analysis: the process in 8 steps
To get a lot of value out of Big Data, you need to take a specific number of steps. These steps help you to structure your project and ensure that you start with a business issue. This is crucial because many projects do not show a return in practice. Usually, a lot of data is collected but hardly analyzed and applied. The figure below shows the 8 Big Data analysis steps and the explanation of how you can achieve success with Big Data:
Figure 2: The life cycle of Big Data analysis
- Identify and define the business issue: here you and your colleagues will explore which business issues are eligible for Big Data analysis. In doing so, first use the most important Key Performance Indicators (KPIs) in your organization or business process.
- Collect and prepare the relevant data: based on the business question, you will select an initial data set and clean it up where relevant. Read more about measuring and improving your data quality here.
- Explore and analyze the data: you are now going to perform a Big Data analysis and explore the data with a BI tool so that you get an understanding of the data and whether it could solve the business issue. You’re also going to visualize the data in a variety of ways. Read more about data visualizations here.
- Put together a definitive data set: you carry out steps 1, 2, and 3 until you have a data set that is good.
- Build the Big Data model: you are going to build a model where algorithms make predictions based on training datasets.
- Validate the model: the model now needs to start being validated by domain experts; they determine if the predictions that the algorithm gives as a result are correct.
- Bring the model into production: if the model is valid, given the initial situation and the business issue and you have the data quality under control(!), then you bring the Big Data model into production.
- Evaluate the results of the model: regularly test whether the predictions of the model still come true and what results it produces. Based on this evaluation, you will create a more sophisticated version of the model that can predict even more accurately.
These 8 steps of Big Data analytics help you to always put a business issue at the center of a technology project and organize the governance with responsible roles (Big Data Governance). In addition, the roadmap makes it clear that it is not a one-time exercise, but an ongoing process of refining and improving the model. Finally, finding patterns in Big Data can no longer be done with traditional analysis tools because the data is too big or too complex. You will have to develop an algorithm such as a neural network (ai) that will do it for you in an efficient and effective way.
From traditional BI to Big Data Science
Traditionally, Business Intelligence (BI) works with structured data that you can store and access relatively easily. You can create cubes or dashboards based on that data. Business Intelligence Big Data Science is about processing (large amounts of) unstructured data and algorithms. How can you process these properly and how will you build a good Big Data analysis? And what else should you be aware of?
A cluster of computers with Hadoop gives enormous computing power
One well-known technology is Hadoop. It provides a framework to access and filter large volumes of data. Hadoop on a cluster of many computers gives enormous computing power. This allows those computers to deliver certain data at lightning speed to the BI tools for the end user.
Big data versus Zero Data
We are firmly convinced that Big Data can add immense value to your organization. However, you should not limit yourself to the possibilities listed so far. Sometimes the data that you don’t record about your customers or processes, the so-called Zero Data, contains an even greater value than Big Data. Curious about exactly how that works? Then feel free to contact us.
Look beyond your own data
It is also advisable to look beyond your own data. Include external data sources and open data in your analyses. In this way, you enrich the internal view with relevant context. Think about demographic (customer) data and market information, competitive analyses, but also things like the weather, traffic movements, or sentiments on social media. These days, you’re more likely to look at problems or opportunities from the outside in, rather than from the inside out.
What does a mature Big Data architecture look like?
The starting point of a good Big Data architecture is that you must be able to analyze enormous amounts of unstructured data just as easily as simple data. In addition, you must be able to easily combine complex Big Data (stored in a data lake) with normal data (stored in a data warehouse). So, in your architecture, Big Data should not be regarded as a completely isolated phenomenon, but rather integrated deeply into various parts of the architecture. The following figure shows a detailed big data architecture.
A mature Big Data architecture like the one above is not something you build overnight, as it involves a significant investment. But this is the dot on the horizon that you will be working towards because all these separate islands with Big Data analyses and applications will eventually become suboptimal or even counterproductive.
Figure 3: The different components of a Big Data architecture with BI tools, a data warehouse, a data lake, machine learning models, a portal, mobile BI, and metadata. Source: Big Data book (2020)
In this architecture for Big Data Science, the data lake deserves special attention. This storage place for Big Data can contain photos, videos, e-mails, sound fragments, sensory data, or other unstructured data. You are going to access and analyze this data with BI tools, these are the Big Data analysis tools.
Follow a 2-track strategy: Big Data Science is more than a Big Data strategy
Of course, you need to start developing policies and a strategy to get Big Data predictive analytics off the ground in your organization, but it is also crucial to start experimenting with Big Data Science quickly. It is a complex field and by trying you will learn and get a much better understanding of the subject matter, the risks, the pros and cons, and the potential returns. A two-track policy, developing policy and experimenting, is therefore recommended. You want to achieve success with Big Data mining and therefore it is good to be aware of the main risks and to anticipate them at an early stage:
- A technology-driven journey: research by IDG shows that more than half of the investments that organizations make in Big Data technology have nothing to do with Big Data applications and the impact of these on processes, ways of working, and people. This ties in with our own experience in practice. Therefore, always start a project from a business perspective and make sure that it is not the technology that is leading, but your business strategy, KPIs, and business processes.
- Complexity and size of the data: photos, texts, machine data, and video images can quickly require terabytes of storage. Although storage space does not cost that much these days, volume remains a concern. Also, because Big Data analysis can quickly become bogged down by the complexity of the data. So, you need a lot of “brute” and smart computing power to set up a good system with which you can develop an application quickly and in an agile. The system must be scalable, future-proof, and testable.
- Data quality: is still a big, underexposed problem in many organizations. Calculations show that around 10% of organizations’ profits evaporate due to poor data quality. With Big Data mining, the challenge of data quality becomes even greater, because a machine learning model that has been put into production often functions as a black-box. Furthermore, in a data lake, there are still hardly any facilities available to measure and improve data quality across the board.
- Ethics & Big Data privacy: when it comes to the processing and analysis of personal data, laws and regulations, such as the General Data Protection Regulation (AVG), it can quickly become quite a roadblock to successfully apply Big Data machine learning. Read more about data privacy law in the Netherlands here, review the ethics surrounding Big Data here, and request privacy Big Data advice here.
Big Data and artificial intelligence (AI) or machine learning on Big Data are two separate fields that have a lot to do with each other. If you want to analyze copious amounts of data without AI, then as a data analyst you might spend years trying to put it all together. If you want to analyze a lot of unstructured data without a machine learning model, the chance of errors is huge, or you will quickly overlook things. And on top of that, AI gets a lot more value because your algorithm can be trained with huge amounts of data. This increases the chance of a reliable and accurate model. The combination of Big Data & AI results in a perfect interplay that increases your chances of achieving remarkable success with Big Data analytics.
Analyzing Big Data is the new gold, the new oil
What if there are a few proverbial nuggets of gold hidden in your Big Data? By which your company knows, for example, a month earlier than your competitor that the price of a commodity is going to rise. Or that the sensor data from an aircraft engine shows that it is having hiccups during a flight, at a certain altitude, and under certain adverse weather conditions. In many cases, engine failure means disaster. It is precisely these kinds of critical applications, but also new business models, that make Big Data enormously interesting. Big Data is therefore also called the new gold or the new oil, because of the great enormous value it represents.
The BI & Analytics Guide™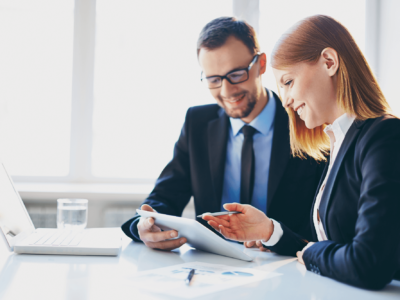
Discover new opportunities and reduce risks with Big Data Management
Or think about the analysis of millions of camera images of psychiatric patients. You can then build a model that allows you to quickly notice abnormal behavior in a patient. Those patterns tell you that there is a high probability that a particular person is “going off the rails” with all the risks that entails. By detecting this behavioral change early, you can perform (additional) checks and controls in a timely manner. That is why organizations are eager to mine that mountain of data, discover opportunities, and manage risks. We would like to help you move from reactive to proactive work based on Big Data predictive analytics.
Big Data solutions and analytics tools
You can only successfully dig up gold or other valuable resources if you select and acquire the right tools, instruments, and solutions. It’s the same with Big Data. You need special Big Data solutions or Big Data analysis tools to store, analyze, and visualize large amounts of data or unstructured data. These Big Data tools fall into three categories:
- Storing Big Data: think Hadoop, MongoDB, Apache Cassandra, and NoSQL, you store the data in a data lake.
- Processing the data: this is an intermediate layer to quickly analyze data regardless of where it is stored in a data lake. Knime, for example, is an open-source environment that is perfectly suited for data integration.
- Analyze, report, and visualize the Big Data: this type of software allows you to dig into the data, perform analyses, and create data visualizations, algorithms, and reports. Examples include Datawrapper, Watson Analytics, and FusionCharts.
There are more Big Data analytics tools available on the market: IBM Cognos Analytics, SAP BusinessObjects, SAP HANA, Microsoft BI & Power BI, Oracle BI, WebFOCUS, Style Intelligence, Yellowfin, Pentaho BI, SAS, BOARD, MicroStrategy, QlikView, Qlik Sense, Sisense, TIBCO JasperSoft, Tableau Software, Infor Birst. We examined all these solutions in our comprehensive BI, Big Data & Analytics Guide™.
Achieving success with Big Data models: 6 characteristics
A successful trajectory with Big Data is characterized by an open company culture that prioritizes learning analytics. And, of course, sufficient commitment and budget opportunities from management. In addition, a great deal of business knowledge, thorough process knowledge, and creativity are required from both the business people and the data scientist. To achieve success with Big Data, a project leader further ensures:
- Alignment with organizational goals and mission: the Big Data goals align with the strategic business vision, so you can achieve your organizational goals. Just building a data lake at random is pretty useless.
- Engaged users: user participation and especially awareness among users of what Big Data can mean for their work, is of significant importance for the success of a Big Data & analytics project. An agile & scrum approach can help achieve that participation.
- Source and data quality: the quality of the data is even more important with Big Data than with regular Business Intelligence projects. With Big Data analytics you are going to make certain decisions automatically.
- Usability and ease of use: the usability, accessibility, and ease of use of a Big Data model must be high.
Solid data infrastructure: the quality and flexibility of the data infrastructure must also be high. You need a robust and scalable system. - A balanced team structure: enough experienced data science experts and a team in which you can align business and IT & BI competencies well. This will enable you to respond better and faster to various information needs.
So how can it be that things still go wrong sometimes? The answer is obvious. Managing the above well and achieving success with big data models is by no means an easy task. They interact with each other and require a steady hand, expertise, and a good dose of experience in the field of Big Data analytics. Request Big Data consulting here.
The Big Data & Data Science Quick Scan
Our Big Data Quick Scan gives you a good idea of where you currently stand in terms of maturity and which steps you can take to increase the added value of your data. Besides content-related and technical issues, we also take a close look at process and organizational embedding. Of course, we do not forget the strategic direction in which your organization is moving. Only then can your Big Data make a strategic as well as a tactical and operational contribution.
The major advantages and disadvantages of Big Data analytics
Advantages of Big Data
- You can do Big Data analytics at relatively low cost (in the cloud) and at high speed
- You can start refining your business model and innovating radically
- You can create endless new opportunities to differentiate, for example by market segment/customer group because you have detailed data at your disposal
- You can make predictive models and, in this way, go from reactive to proactive. This has a positive effect on your profitability and customer satisfaction.
Disadvantages of Big Data
- It is complex because you have to combine it with artificial intelligence and machine learning. The knowledge of Big Data machine learning is very scarce. The Data Scientist is a jack of all trades.
- Privacy issues can easily throw a spanner in the works, processing personal data without a legally valid basis is asking for big problems.
- An enormous amount of money is wasted, because only a small proportion of the initiatives are put into production. Estimates range from 10-15%.
Big Data Analytics success stories
More and more success stories about Big Data & analytics are surfacing at a rapid pace. These stories also no longer go unnoticed in the media. The fact that the Amsterdam fire department uses Big Data to prevent fires has already made it to the NOS evening news and the BBC. That the Amsterdam police can catch crooks before they commit a crime entitled them to a podium place in ‘The Smartest Organization in the Netherlands’.
Figure 4: These are some of the advantages of Big Data Analysis simplified
The fact that the city of Dublin optimizes its traffic flow with Big Data is a shining example for all public institutions. They now better understand that you can greatly improve the service to citizens. In short: these success stories convincingly show that Big Data Predictive Analytics can make the difference between stupid and smart organizations. Between the losers and the winners.
Want to become a smart, data-driven organization too?
Then feel free to contact us for an exploratory meeting with one of our Big Data specialists. We would be happy to help you get your organization working in a data-driven way. We make your Big Data analytics applications successful.
About Passionned Group
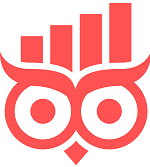