What is artificial intelligence (AI)?
Artificial intelligence, what is it now? The search for a simple artificial intelligence explanation is difficult. Our artificial intelligence definition reads as follows: “the development and application of (self-learning) computer algorithms that are independently able to discover existing or new connections and/or patterns in (big) data and independently make decisions, thereby dramatically improving the effectiveness and efficiency of a process or business processes.”
Figure 1: What is the impact and scope of artificial intelligence?
Government agencies, consultants, and vendors all use their own AI definitions and use AI jargon within their own organizational context. In Figure 1 we give an overview picture of the different AI applications and their impact on our actions and our thinking, learning, and decision-making process. One thing is certain: AI changes everything.
Artificial intelligence meaning
Artificial intelligence (AI) is an applied science in which computers take over certain human tasks, such as learning, reasoning, analyzing, solving problems, and making decisions. According to the compilers of the National AI Course, artificial intelligence takes on meaning because algorithms are increasingly able to handle cases or decision-making processes independently. According to them, one speaks of AI when a system can independently perform tasks in complex environments, without constant guidance from the user or trainer.
Artificial intelligence in business
Around AI companies and AI technology is an atmosphere of optimism (“AI is going to solve all the big world problems”), but at the same time a haze of secrecy (“are robots going to take over everything and dominate our lives and work?”). Artificial intelligence companies are sexy and can quickly count on high ratings from stock market analysts and potential investors. This while the technology of artificial intelligence companies has often not even crystallized yet and a stock exchange listing is still a long way off. Is artificial intelligence overvalued today? Is AI possibly overhyped? To what extent is artificial intelligence about IT? How can organizations develop a responsible AI-first strategy without unnecessarily throwing money down the drain? But also: how can they avoid missing the AI boat, leaving commercial opportunities and possibilities of artificial intelligence underutilized?
AI science
“Is artificial intelligence data science and thus a part of the field of Business Intelligence (BI)? And what does the term AI science actually mean?”, people ask themselves. Instead of BI, there is indeed a lot of talk about data science, data-driven work, and information-driven work. These terms are more or less interchangeable. Data science or AI science often emphasizes the statistical elements of BI such as artificial intelligence, text mining, machine learning, and statistical models. Information-driven work emphasizes process and control. BI is then the umbrella term that encompasses all of these. The 7th edition of the book The Intelligent, Data-Driven Organization explains all the relevant terms in detail in the context of this definition discussion.
What can an AI system do?
An artificial intelligence system is capable of growing into a fully autonomous, self-learning system in any organization, where the algorithms drive the decision-making process and make decisions independently. In other words, the human being decides and the algorithm decides. Behold the essence of an operational AI system. This AI process may appear overwhelming and threatening, but in the background, there is still room for humans as supervisors. Humans keeps an eye on the process. They are responsible for the outcomes of the automatic decisions and ultimately for the explainability of the algorithm. People talk about Explainable AI (XAI). In other words: how does the algorithm arrive at its decision? This implies that humans remain responsible and accountable and must always be able to explain how artificial intelligence and in particular the algorithm arrives at a particular outcome.
What are the most important AI trends of 2024?
Every year Passionned Group publishes what it considers to be the most important trends, including a number of big data artificial intelligence trends. From the collected AI trends 2024 we can conclude that AI technology is becoming more and more accepted globally, but that AI applications are followed more and more critically by the media, politicians, citizens, and other stakeholders. This critical attitude towards AI has everything to do with the inscrutability, contestability, and explainability of the algorithms that are attached to artificial intelligence applications.
The Artificial Intelligence handbook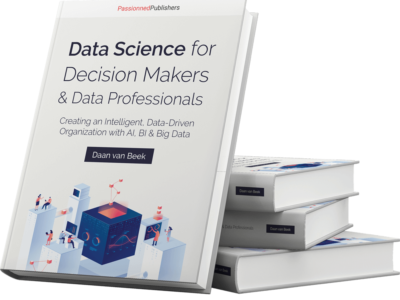
Artificial intelligence trends of 2024 at a glance
The most relevant artificial intelligence trends for organizations can be summarized as follows (not exhaustive):
With Robotic Process Automation (RPA) and Artificial Intelligence (AI) administrative business processes in finance, logistics, and other sectors will become increasingly automated. One speaks of hyper-automation in this context.
- Artificial Intelligence is growing into a powerful weapon in the fight against fraud and organized crime, from credit card fraud to money laundering.
- AI-driven information security, or ensuring cybersecurity such as detecting malware, phishing, and fake news, is also a trend that is sure to continue.
- Artificial intelligence will increasingly be used successfully to further streamline the IT department and secure systems. Think of predicting downtime, taking over human tasks, and automatically resolving issues through self-healing and self-correcting systems (predictive maintenance artificial intelligence).
- Artificial intelligence will make strong inroads in call centers and in customer care and customer departments of organizations, both in retail and in financial and business services. Virtual assistants and chatbots will take up a large part of the workload.
- In recruitment and selection and ongoing application processes, human resources departments will increasingly use AI technology to screen and assess candidates (pre-screening of applicants and automatic resume screening).
- The use of AI will begin to make a significant contribution to the quality of healthcare in a wide range of areas. With AI technology, you can make diagnoses automatically, prescribe the right medications automatically, and build prediction models for disease states.
- The application of artificial intelligence is not limited to hospitals. The mental health sector also sees the usefulness of AI applications. For example, robots will increasingly be used to care for people with dementia and to combat loneliness in the elderly.
- Artificial intelligence will affect the retail sector in several ways. It can improve the shopping experience by personalizing it to a great extent. Algorithms will provide reliable demand forecasts, minimum physical inventories, and optimal, dynamic pricing strategies, among other things.
- Artificial intelligence will also make its appearance in education. Adaptive learning tools that enable independent, personalized learning are already being applied. In addition, prediction models will support students in putting together the right subject package.
- Artificial intelligence in logistics will take off in a big way. The application possibilities are numerous and vary from autonomous driving vehicles and robots for order picking, smart route planning, and Internet of Things implementations for containers to predictive models for demand, orders, and delivery times.
Different types of artificial intelligence
In the literature on the different types of artificial intelligence and the AI models we can distinguish, there is a broad agreement that there are roughly three types of artificial intelligence (see Figure 2). In English, they are known as Artificial Narrow Intelligence (ANI), Artificial General Intelligence (AGI), and Artificial Super Intelligence (ASI). The weak variant, also known as the “short-sighted” variant, is only capable of performing one task efficiently (think of a game of chess or Go). The broader variant, AGI, is capable, like humans, of abstract reasoning as well. As a result, this variant is able to respond to unexpected situations (improvisation). The strongest variant, the super variant AIS, transcends human intelligence. At the time of writing, it is still unknown what the super variant will eventually be capable of.
Figure 2: The different types of AI
In addition to this rough classification, there is another global classification of the different types of artificial intelligence. For example, within the field of artificial intelligence, Kaplan and Haenlein distinguish three different types of AI systems: the analytical, the human-inspired, and the humanized form of artificial intelligence. The analytical variant has some cognitive properties that make it capable of learning. The human-inspired variant possesses emotional intelligence in addition to cognitive properties, and the humanized AI variant is also socially intelligent and can interact with others in addition to communicating.
AI and machine learning
AI, data science, machine learning, algorithmization, data science, artificial intelligence and AI automation are used interchangeably in everyday speech without users being aware of the (exact) definitions. This Babylonian confusion of tongues muddies discussions and can even cause sentiment around AI and machine learning to shift and the benefits artificial intelligence has to offer to be underplayed. To better understand AI, here are a few more terms you absolutely must know:
- Data science is the overarching field that deals with processing and analyzing large amounts of data, and/or unstructured data.
- Machine learning is a field that lets computer algorithms learn without you having to explicitly program them.
- Deep learning is a specific form of machine learning where algorithms learn from (large amounts of) data.
- An algorithm is a mathematical formula. In programming language, it is an instruction, a piece of code, to solve a problem. The algorithm is a (computer) program that, based on (historical) input, arrives at an analytical model that you can use to assess new cases.
Artificial intelligence applications
What AI applications are there? Artificial intelligence applications, or AI applications for short, can be roughly divided into a number of main categories, depending on the technology on which the artificial intelligence software is written.
Natural Language Processing
Natural Language Processing (NLP) or speech recognition: the field that deals with the reading, understanding, and production of human language by computers. This is where a number of fields come together such as AI, computer science, and computational linguistics. Speech recognition is a specific application of AI that recognizes speech and can convert spoken words into typed text. NLP is particularly used by virtual assistants and chatbots in call centers and customer departments to replace human communication.
Data mining
Data mining: finding connections, patterns, and correlations in structured data using machine learning, statistics and database techniques. The goal is to gain new insights that are “hidden” in the data and to acquire new knowledge.
Text mining
Text mining: finding connections, patterns and correlations in unstructured data such as text. Again, the goal is to gain new insights and knowledge.
Image and face recognition
Image and facial recognition: an AI application that is gaining ground following China’s lead is facial recognition by intelligent cameras. In public spaces such as streets, airports, and buildings, facial recognition is increasingly used for surveillance and detection purposes. Computer vision allows you to mimic and surpass human vision. This AI application aims to use computers to identify objects and people in images and videos.
The AI process in 15 steps
You can divide the AI process into a number of logical steps by analogy with the BI process. Only now the human doesn’t make the decision but an algorithm pre-cooks the decision (see Figure 3 below).
Figure 3: The process made specific to developing algorithms that pre-cook or make decisions.
- The first three steps are all about the collection, filtering, and combining of the AI data.
- During steps 4 to 6, you will not aggregate the data but analyze, visualize, and interpret it. Because it is in the many details that you will probably find connections, certain patterns. For structured data, you can use a BI tool or a data discovery tool. For unstructured data like e-mails and photos, you will have to explore the data with other tools and techniques.
- Based on training data and test data, you will build, fine-tune, validate, and test the machine learning model during steps 7 to 9. For each type of algorithm, you have to deal with different scenarios here.
- During step 10 the focus is on enriching the AI model. The machine learning model itself will of course no longer be modified at this point, as it is finished.
- The enriched machine learning model is now ready to be put into production and the algorithm must remember the values determined after the training (see steps 10 and 11). “Remember” here means “preserve”. This is how you will need to store a model, otherwise you will have to train it again every time you want to assess a new situation.
- Step 13: The algorithm independently makes or pre-cooks a decision. For example, the algorithm decides to price out certain foods in the supermarket because the shelf life is about to expire, or the algorithm increases the price of airline tickets for high-demand destinations.
- During step 14, you will spread new insights gained during the AI process further into the organization so that everyone can take note.
- Step 15, anticipating change, speaks for itself, but it’s a very important step.
The economic significance of artificial intelligence
A lot of money goes into the AI business and into AI companies. Good software developers and data scientists are scarce and therefore expensive. Venture capitalists, banks, and private investors are collectively pumping billions of euros and dollars into startups that are developing new products and services based on AI applications. AI applications are finding their way into almost every industry and sector. The listing in Figure 4 is by no means complete, and the number of applications is likely to continue to grow.
Governments are also making a significant financial contribution because they recognize that artificial intelligence can make a significant contribution to public safety, better healthcare, streamlined infrastructure, energy savings, better quality education, and so on. The promising concept of the smart city and a more sustainable society will hardly get off the ground without artificial intelligence and big data, they realize. These are advantages artificial intelligence brings to the table and they make a case for considering a so-called AI-first strategy in any new project or initiative.
Figure 4: The AI applications broken down by industries.
The added value of an AI-first strategy
With an “AI-first” strategy, embed artificial intelligence (AI) in everything you do and do so in all your business processes. AI-first means that in everything you do you look at whether algorithms can improve (accuracy) or speed up (turnaround time) the decision process and the decisions themselves. This requires a totally different organizational culture than when you start developing reports or KPI dashboards. Perhaps an algorithm will even make reports obsolete.
Artificial intelligence pros and cons
The artificial intelligence advantages and disadvantages are widely reported in the press on a daily basis. The consultants of Passionned Group are at all times prepared to guide entrepreneurs in the AI process and to give form to the aforementioned AI-first strategy, with the goal of making the most of the advantages artificial intelligence offers and avoiding and/or limiting the disadvantages of AI. Read more also about the 25 key benefits of Data Science here.
Disadvantages of artificial intelligence
The disadvantages of artificial intelligence, especially for individual workers, citizens, consumers, and other stakeholders, are also widely reported in the press on a daily basis. Discussions about the potential negative consequences associated with the application of artificial intelligence and algorithms in the public domain focus in particular on the fundamental rights of citizens.
Advantages of Artificial intelligence
The artificial intelligence benefits for companies and institutions seem at first to be primarily in the area of labor savings: robotic process automation (RPA) and the deployment of physical robots in industry to replace flesh-and-blood employees. But by seeing artificial intelligence as merely a tool for saving on labor costs, you sell the concept short. Provided it is applied correctly, you can take stock of the benefits of artificial intelligence for your industry and organization from four different perspectives (people, organization, society, and nature) (see Figure 5).
Figure 5: The benefits of artificial intelligence.
Organizations can also use artificial intelligence to better predict demand, optimize their sales channels, improve the (preventive) maintenance of machines, increase production and quality, personalize services, and improve customer experiences. In general, algorithms can significantly accelerate, improve, enrich, and even partly take over the entire decision-making process in organizations.
7 blessings of artificial intelligence
Below we present seven concrete artificial intelligence examples. They are concrete, practical AI applications that illustrate the blessings and added value of artificial intelligence. The list is by no means exhaustive.
- Drones equipped with artificial intelligence detect gas leaks, inspect dikes and agricultural and horticultural crops, and determine fire, storm, and roof damage for insurers.
- Machine learning and AI data support hospital radiologists in analyzing X-rays and determining radiation plans for cancer patients.
- Thanks to predictive policing, license plate recognition via smart AI cameras, and the deployment of robotic dogs, police are better prepared and criminals have a better chance of being caught.
- Banks, insurers, and benefit agencies use algorithms, artificial intelligence, and data science to detect potential fraud.
- Thanks to algorithms and artificial intelligence software, supermarket owners are better able to match supply and demand for fresh produce in their fight against food waste.
- Regional power grid operators can save a lot of costs by deploying AI technology for smart maintenance and quickly pinpointing the cause of power outages.
- In the fight against drug smuggling, an AI-driven risk analysis in the port of Rotterdam determines which containers will be checked and which will not.
7 pitfalls of artificial intelligence
Below we also identify seven pitfalls that can occur when applying artificial intelligence. They are concrete AI applications from the real world. A number of “accidents” with artificial intelligence and big data can be considered “teething problems” or learning processes. A wise lesson: fail before you start! Again, this list is by no means complete.
- Microsoft once launched a chatbot that turned into a true racist in 24 hours. The cause was a troll army on Twitter that fed the self-learning chatbot with a flood of racist and sexist messages.
- Several government agencies use algorithms, big data, and artificial intelligence to assess fraud risks in benefits and taxation. In some cases, this led to obvious discrimination and privacy violations.
- The U.S. saw the first fatal accident involving a self-driving car equipped with artificial intelligence, an uber. The prediction algorithm missed the movement of a female pedestrian in the dark.
- A fire broke out in a British distribution center of an online supermarket chain after three robots collided in the automatic process of order picking.
- A well-known Chinese businesswoman’s face was pictured on the side of a city bus. When the bus pulled up, facial recognition software incorrectly identified her as a pedestrian and considered her a traffic offender.
- Facebook discontinued an experiment with artificial intelligence after two speech robots developed their own language that only they understood.
- In algorithm-driven stock trading, so-called flash trading, sometimes the algorithm goes haywire, leaving everyone with their hands tied. The moral of the story: too much dependence on artificial intelligence makes you vulnerable.
AI applications and the infringement of fundamental rights
Surveillance by governments can violate fundamental rights and privacy rights in particular. Relational privacy, the right to personal autonomy and human dignity may be at stake with far-reaching robotization at home, in healthcare, or at work. The use of algorithms and AI data in job applications may violate the principle of equality. The right to be forgotten may be violated in marketing and promotional campaigns. Algorithmic censorship in social media may violate the right to freedom of expression. Application of algorithms in the administration of justice (“the robot judge”) may limit the right to an effective remedy and access to an independent and impartial judge and undermine the right to a fair trial. Biases in AI data in government decisions can impair the right to non-discrimination by adding biases. And in the case of algorithm-driven government decisions, citizens’ right to transparent and supportable reasoning cannot be easily overridden.
NOTE: all of these drawbacks in the application of artificial intelligence and algorithms can occur, but by proceeding carefully, you can avoid reputational damage. With sufficiently strict safeguards and tools such as anonymization and pseudonymization, and so on, the adverse effects can indeed be prevented or limited. You have to take your time and seek advice, but that often does not happen.
AI and ethics: Go in depth
Passionned Group has put together two articles on AI and ethics that you can use to explore this subject in depth. The first article on AI ethics focuses on the 10 commandments of computer ethics. The second article describes 5 sacred principles for responsible application of artificial intelligence.
- The ethics of Big Data and AI: temptation and fear
- Data ethics: the 5 sacred principles for responsible application of AI
Future development of artificial intelligence
Future AI applications are dominated by increased surveillance and standardization. While the government constantly points out the opportunities and possibilities that artificial intelligence and algorithms offer to ambitious and innovative organizations, it is also mindful of the risks of AI. When algorithms or artificial intelligence are deployed in the wrong context, in the wrong way, or for the wrong purposes, the impact can be enormous, they warn. At the same time, the government sees opportunities to use AI applications to address social problems. From this dilemma, standardization and supervision will remain a spearhead in the coming years, not only nationally but also in a European and UNESCO context.
Hiring an AI specialist?
Have you already followed our Artificial Intelligence training and do you want temporary support in setting up an AI project in your organization? Are you struggling with the question of how to use AI to make your organization work in an information-driven way and do you want objective advice on this? Are you looking for an Artificial Intelligence specialist, an Artificial Intelligence expert, or an AI interim officer? Please contact our Manager of Operations & Recruitment.
Or do you also want to become more familiar with the principles of artificial intelligence, and the AI process and enjoy all the benefits of AI applications? Do you want answers to the question “is artificial intelligence possible for our company”? Are you struggling with your recruitment artificial intelligence vacancies? Get in touch with us.
Order the AI book now
Books on artificial intelligence abound. However, there are few artificial intelligence books that are conceptually sound and at the same time have a not-too-technical angle. The book The Intelligent, Data-Driven Organization has that practical angle. This Artificial Intelligence book is available in both Dutch and English. Order the book now directly.
About Passionned Group
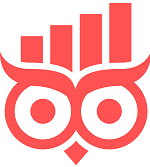