Avoid the biggest data science pitfalls
Over the past two years, the teachers of the Master of Data Science masterclass have taught dozens of learners the essential principles of data science. The experiences, feedback, and personal ambitions of our students have painted a fairly consistent picture of the data science issues plaguing the workplace. Collating all of their feedback, we’ve distilled eight data science success factors that we’d love to share with you. These factors help businesses streamline data processes and achieve more reliable, actionable insights. By focusing on these success factors, organizations can transform data challenges into opportunities for growth and innovation. The key take-away: let the data work for you instead of vice versa.
1. Don’t be blinded by the hype
All over the world, governments, the corporate world, and universities try to one-up each other when it comes to the question of who is investing the most in data science and the key technology – Artificial Intelligence (AI). The peak of inflated expectations has been reached and the hype is at an all-time high.
Take the Dutch government, for example. Secretary of economics Mona Keijzer is repping a Strategic AI Action Plan and talking about investing 2 billion euros in AI over the next 7 years, to be raised by the government and corporations. The vagueness of these plans makes these announcements feel like a case of the emperor’s new clothes, though. Meanwhile, business managers in organizations are grappling with theoretical and practical issues. How does data science relate to machine learning and AI, for example?
2. Determine your organization’s ambition and maturity level
A baseline measurement is a proven method of determining how the various disciplines in the organization view data science. It’s usually the first step in the improvement and change process: where are we, as an organization, on the “data science ladder”?
Take your organization’s temperature. To what extent are people open to new insights and technologies? Is there a (shared) vision of the future? Is leadership thinking in terms of scenarios: if we ignore data science, what risks do we run? Is there an improvement mentality and the desure to deliver predictable processes and results? Is data seen as the most vital asset? Is there a realization that data-driven working and management can grow into becoming the new normal? Or are we just following hypes? Is solving problems considered more important than preventing them? Is there a fear of the unpredictable and undesirable outcomes of data science projects? Are people data literate? Is the IT living up to its advisory role?
3. Make data quality your absolute top priority
Subpar data quality is an important reason for organizations to put off or completely abstain from starting up data science projects, according to our Master of Data Science trainers.
Rationally speaking, this reticence can be easily explained: garbage in, garbage out is a well-known big data pitfall. That aside, organizations are struggling with data of all kinds: structured, unstructured, semi-structured, master data, metadata (data about data), sensor data, weblog data, unprotected Excel sheets, subjective data such as NPS scores and FeedbackNow data, and on and on. If you dump all of that data into a data lake, safeguarding the data quality becomes an impossible mission.
The big question is: what can we, as an organization, do with the enormous mountain of big data at our disposal, and how do we turn raw data into valuable information?
There’s an impression that organizations use subpar data quality to justify avoiding difficult topic like predictive algorithms, genetic algorithms, and optimization algorithms. People tend to see these as black boxes. Nevertheless, having access to clear information and good data quality (up-to-date, unique, consistent, complete, integer, and logical) is of fundamental importance to excellent external services. But you need more for successful data science: internal support.
4. Introduce data science within a wider framework of data-driven working
Creating support for new technology in organizations and promoting user acceptance have always been hot-button issues. Business Intelligence as a discipline still has to prove its worth on a daily basis, in traditionally organized organizations. As such, it’s smart not to introduce data science as an isolated project in the organization.
I want to understand how data science works, know which tools are available, and learn how to use data science (tools) to generate better management information.
Frame it within a mission as broadly appealing and inspiring as possible. For example, it’s Google’s mission to organize all the information in the world and make it universally accessible. Facebook originally wanted to empower people to share more and make the world more open and connected. Both Google and Facebook can be criticized plenty for how they’re trying to achieve their mission; their algorithms and methods have caused international controversy, despite their clear mission statements. For organizations closer to home, the concept of data-driven working or data-driven management is a fine umbrella to hang their own mission under.
5. Always strive for a compelling business case
Business managers, commercial directors, and marketing managers want to know how data science can lead to a competitive edge, while IT managers, enterprise architects, security professionals, PR managers, and lawyers have entirely different concerns when it comes to data science. And the data scientists themselves mostly want to experiment endlessly without disruption, to find interesting patterns and make algorithms, unhindered by financial KPIs (examples) like pay back periods, return on investment, legal frameworks, and illegible behavior codes.
Admittedly, this is somewhat exaggerated, but data science can’t escape the need for a convincing business case, a future-proof business model, and an interesting revenue model. The times of high burn rates, or negative cashflows, being hot are far behind us.
In short: first, try to determine to which problems data science offers a solution. Look for the underlying basic needs and try to formulate it as clearly as possible. Take stock of the required time, resources, and capacity.
6. Combine all the knowledge and experience in a Data team, Data lab, or Data hub
As soon as data science becomes serious business, it’s time to start looking for potential synergistic benefits. Scaling up activities, recruiting data science talent, and sharing knowledge are all realistic options.
Many of our trainees indicate that they’re faced with a very small labor market. Data scientists are a rare breed, and thus costly. Nevertheless, organizations are looking for possibilities to give data science a more or less formal status around the margins of the organizations. Broadly, you might see this as something like the formation of a Data team, founding a Data Lab, or starting up a Data Hub.
- Setting up a Data team is a touchy subject. Avoid a homogeneous culture by making your team diverse.
- A Data Hub is a modern, data-centric architecture for storage, according to a well-known storage provider. The Data Hub supports analytics and AI by enabling companies to consolidate data and sharing it in the data-first world we’re living in nowadays. In contrast to a data lake and traditional DAS architectures primarily developed to store data, a data hub was developed to share data.
- The city of Amsterdam, for example, chose a highly practical approach. In Amsterdam, a data lab is a place of work, a knowledge center and an open podium for data professionals and anyone interested in data. A place for smart, innovative, and careful use of data.
I want to use the skills and knowledge gained in this workshop to convince the organization of the use and necessity of a data science project.
However you embed data science in the organization, sharing data is a trend, even in the government.
The Business Intelligence book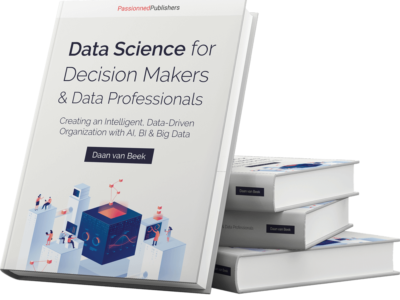
7. Study the ethical framework and apply the FAIR principles
FAIR stands for: Findable, Accessible, Interoperable, and Reusable. Another initiative is the Personal Health Train, a metaphor for the agreements, architecture, and implementation of the responsible use of health data in AI applications. The PHT builds on the FAIR data principles. Citizens, patients, healthcare professionals, or researchers drive the “trains” (algorithms) to ask questions to “stations” (data sets) and get answers. The most important concept of the PHT is that data isn’t brought to the algorithm, but the algorithm to the data. The data stays at the source, while algorithms can still learn from them.
All trainees will sooner or later be faced with the ethical issues associated with data science. Worldwide, dozens of codes of conduct and principles have been published about it at this point. Although they’re usually drafted with the best intentions, there is often a lot of overlap and sometimes they’re little more than window dressing.
8. Use data science to jump-start your own career
Why would you risk tangling with something as complex and sensitive as data science? The personal goals expressed by our trainees at the start of our Master of Data Science training course answer this question of conscience, giving us some enlightening insight into the ambitions of the trainees.
The thirst for knowledge is shared among all the trainees, but they also unanimously want hands-on experience with data science and the available BI tools (“which tools can we use to make data work for us”). Trainees also struggle with their own role in the organization. They want to know how to build up a Data team (“how can I recruit the right people with the right competencies”), but at the same time they want to become a “linking pin between the business and IT” and be taken seriously by management (“I want to have enough experience to be a good conversation partner on the various levels within the organization”).
I want to function as the missing link between the business and IT
Some trainees have a mission like “convincing the organization of the use and necessity of a data science project”, or “selling data science internally by promoting it”, or “creating the right mindset for big data and data-driven working”. Although most trainees put the needs of the company first, some are also honest about their own goals: keeping their CVs future-proof.
Conclusion
In short: learning about data science will quickly give you an edge. Suddenly, you are the expert in the organization, giving your career an enormous boost. That’s not only good for your (future) employer, but also for yourself. Do you want to learn all about data science? Enroll in our Master of Data Science masterclass.