The latest business trends and technology trends: a fresh perspective
After a challenging year for many businesses (not to mention governments and individuals) and an economy straining to preserve itself, many organizations are now surveying the landscape. What new investment opportunities have presented themselves, and which business trends or technology trends should they definitely take advantage of? Employees and governments are also refocusing: they might choose an entirely new career and/or invest in (re)training. Think in opportunities and new perspectives will present themselves. Passionned Group has, as usual, charted the most important business trends and technology trends while offering all the support an organization needs to be able to face the future. While this list is not exhaustive, we’ve identified the following seven business and technology trends for 2021 that organizations and individuals will definitely have to consider.
Trend 1: The rise of the Internet of Behaviors
The global rollout of the Internet of Things (IoT) and 5G is still in full swing. While billions of devices are still being connected, the contours of a new network are beginning to reveal themselves: the Internet of Behavior (IoB).
According to Gartner, the Internet of Behavior seamlessly connects to the IoT. The essence of the IoB is the use of (big) data analytics to affect the behavior of citizens. To that end, the activities of billions of people will have to be monitored worldwide. The expectation is that by the end of 2025, over 50% of the world’s population will already be “caught” in one or more IoB applications. While the IoT was mostly seen as a positive (predicting machine outages, providing remote healthcare, helping with precision agriculture, et cetera), the IoB is looking a bit more dystopian. The IoB could spell the end for the last remnants of our privacy. Every activity and movement of billions of people could be followed within the IoB, so goes the doomsday scenario.
Tracking is the new normal
Tracking GPS data, automatically registering driving habits and purchasing and payment habits online, using computer vision to see if health protocols are being followed, and so on – tracking is becoming the new normal. China is the front-runner when it comes to the IoB. Using AI-based facial recognition software and hundreds of millions of cameras in public spaces, the Chinese government is attempting to influence the behavior of its citizens through mass surveillance. Whether or not the term IoB is here to stay remains to be seen. One thing is certain: discussions about privacy, human rights, (self)censorship, the power of social media platforms, and freedom of speech will only become more intense. Smart entrepreneurs will keep this in mind when considering their technical innovations and outward communications.
The BI & Analytics Guide™ 2025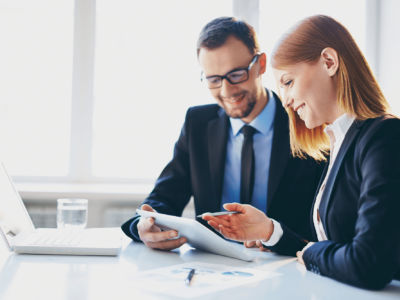
Trend 2: AI is becoming commonplace while facing increasing scrutiny
Artificial Intelligence (AI) is becoming more prevalent in public spaces, business, healthcare, and within the government. The number of success stories has risen beyond count and they’re often widely covered by the media. “AI will eradicate world hunger”, “algorithms accurately predict flu trends”, and “model predicts the chance of criminal recidivism”. The promises of artificial intelligence are great. But the data regularly proves to be unreliable. It’s time for a reality check: AI isn’t an end unto itself, but a means to an end.
Public opinion regarding AI is beginning to turn
Various consumer organizations, supervisory bodies, and governments are becoming more critical of the dark sides of AI in general and algorithms in particular. San Francisco, for example, banned the use of facial recognition software in its battle against fraud, while New York City wants to rein in the use of algorithms when screening job applicants. Other states are considering following their lead. The Australian Taxation Office retired its controversial Robodebt system. The debate surrounding the ethics of AI is getting louder and louder.
AI is here to stay
Regardless, organizations that don’t explore the possibilities and limitations of AI are going to be left behind eventually. AI is here to stay and will become increasingly closely integrated into business processes and workflows. Examples range from algorithms that detect aneurysms, deep learning systems that can determine the aggressiveness of prostate cancer more accurately than most pathologists, software that determines when a patient can leave the hospital, and epilepsy patients that benefit from so-called AI implants.
But there are countless examples outside of healthcare, too. AI can predict the harvest in greenhouses. Algorithms determine the credit score of citizens who apply for loans. Big data machine learning predicts students’ test results. Factories automate visual inspections using video algorithms to detect corrosion in planes, ships, and machines and more accurately inspect gearboxes, pumps, heat exchangers, cavity walls, and safes.
Trend 3: Value-based healthcare is more relevant than ever
Zooming in on healthcare, we can see that Value-based healthcare is a concept that healthcare providers can no longer afford to avoid. Value-based healthcare is not a popular topic among the general public, but European countries can’t avoid taking this concept seriously now that it’s been popularized in the USA. Much has already been written about the impact of COVID-19 on Value-based healthcare. Most analysts in the healthcare industry expect COVID-19 to function as the catalyst for a wider discussion on this topic that will lead to its further adoption. The booming costs of healthcare, capacity issues in hospitals, a lack of healthcare professionals, and the ongoing waste problems in this industry all contribute to this trend.
Central to the concept of Value-based healthcare is the term “patient value”. The definition of patient value is: the relevant health outcomes of the care provided to the patient divided by the costs per patient over the cost of the entire care cycle. As a formula: value of provided care to patients = health outcomes/care costs.
Ethical dilemmas rear their heads
Discussions about Value-Based Healthcare often turn into discussions about medical ethical dilemmas, as can often be seen on social media. But these emotionally charged discussions don’t always do justice to the rational VBHC concept as envisioned by Michael Porter. More serious are the discussions that happen within professional spheres, the healthcare industry, and democratic bodies. Within some government bodies, discussions about triage and who gets what care and when are raging on, and these tricky topics will no doubt keep popping up in 2021.
The Data Science book for Decision Makers & Data Professionals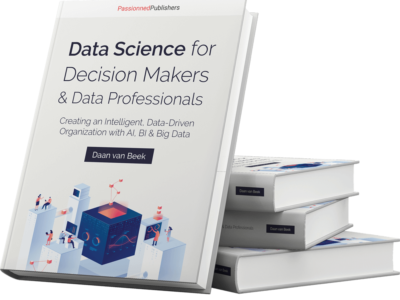
Trend 4: the demand for real-time data is greater than ever
Since the outbreak of the pandemic last year, the demand for current, reliable, and especially open data has massively increased. The general public is regularly confronted with “corona dashboards” displaying data that is, by definition, lagging behind the current situation. Meanwhile, the demand for real-time data is increasing in healthcare institutes and society in general. Suddenly, everyone is a data expert and/or virologist embarking on a journey of data discovery: the iterative process of recognizing patterns and peaks in large volumes of data.
Data literacy remains relevant in 2021
Data analytics, as a practical application of the wider topic of BI & data science, is a typical example of a field where one starts by following a training course. You’ll have to clock a lot of hours before you have any credibility. Correctly interpreting and reading out a dashboard (example) is an art in and of itself. Data literacy is as relevant as ever, in other words. Real-time analytics, including so-called live dashboards, are going to take off, partially thanks to the Internet of Things, sensor technology, and 5G (see also trend 7), and despite the sometimes confusing technology. Dashboards are becoming increasingly important because visualized information is easier and faster to process for the human brain.
In his book “Data Science for Decision Makers”, author Daan van Beek clearly explains the relationship between these terms. Instead of BI, many people speak of data science, data-driven working, or information-driven working (especially in the public sector). These terms are more or less interchangeable. Data Science often focuses on statistical elements such as artificial intelligence, text mining, machine learning, and statistical models. BI (Business Intelligence) focuses on using data to inform decision-making. Information-driven working emphasizes the process and management. Data Science can be seen as an umbrella term that encompasses all of these aspects.
Trend 5: Everything-as-a-service is becoming the norm
In his book Data Science for Decision-makers, author Daan van Beek describes the Cloud-first principle, in addition to the AI-first and mobile-first principles. If possible, host all your IT systems in the cloud. Software-as-a-Service, or SaaS BI, is elastic, reliable, and scalable. You pay for the capacity that you need at any given time.
The trend of offering IT services as cloud services has been going on for decades. The roots of this development can be traced back to the 1960s, when IBM and other mainframe providers introduced the concepts of time sharing and utility computing, the predecessors of ASP (Application Service Provider), and cloud computing.
SaaS is your best friend
Cloud computing as a trend is going to affect the existing business intelligence market and related fields for the foreseeable future. Providers will keep introducing new, “innovative” products and services to surprise and unburden organizations, such as Database as a service, Data lake as a service, Governance as a service, ETL as a service, Data Mining as a service, Data management as a service, Process Mining as a service, Security as a service, Privacy as a Service, Blockchain as a Service, and so on. Or, as a BI tools vendor recently put it: SaaS is everyone’s new best friend.
Edge computing facilitates the IoT
The new star in the firmament is the so-called distributed cloud, which develops off of existing concepts such as the public cloud, the hybrid cloud, and edge computing. Distributed cloud is the distribution of a public cloud across various physical locations, while the cloud provider remains responsible for every aspect of the cloud service: architecture, delivery, operations, management, and updates. Data centers can now be placed anywhere. Edge computing processes data generated by IoT devices as close to the source as possible, on the edge of the network.
This year, vendors will continue to introduce new terms that play off the cloud computing megatrend. It’s essential to stay critical: how solid is their management? Are you getting more bang for your buck? Are the certificates valid? How is their security? What’s the server uptime? And so on.
Trend 6: Hyperautomation is the future
Hyperautomation is based on the idea that you should automate every process that can be automated. Organizations lose a lot of time to fixing technologies and patching systems that are not Lean and don’t function optimally. Unconnected, contaminated systems and (internal) business processes that are not explicitly described also cause their fair share of trouble.
COVID-19 provides a boost to the digital transformation
Organizations that don’t invest in hyperautomation are putting themselves at a disadvantage. COVID-19 caused the digital transformation to leap forward seven years, according to a McKinsey study. The hyperautomation approach is about identifying and automating as many business processes as possible using various techniques that seamlessly work together, such as Artificial Intelligence (AI), Machine Learning (ML), Natural Language Processing (NLP), Robotic Process Automation (RPA), advanced analytics, and business process management (BPM).
The goal of hyperautomation is making more AI-driven decisions, completing another step of the digital transformation. Daan van Beek, author of the book Data Science for Decision-Makers says: “Automate what can be automated. Ensure loose coupling and maximum cohesion in your systems and processes. That’s how you create a more flexible organization while keeping your options open for future improvements. If possible, use simulations in order to safely experiment.” Gartner analysts predict that hyperautomation could lower the operational costs of organizations by an average of 30 percent in 2024.
Trend 7: 5G rollout continues unabated
The international trade association of telecom providers expects that the 5G rollout, after a minor setback in 2020, will continue in 2021. In a report, the GSM Association indicates that despite the delays caused by the pandemic, the 5G rollout is back up to full speed: early this year, 113 telecom providers in 48 countries had already rolled out 5G networks.
Indoor coverage still leaves something to be desired
The possible applications of 5G are legion. Self-driving cars and delivery drones may be among the most exciting examples of 5G applications. But precision agriculture, remote healthcare, augmented reality in industry (inspections and preventative maintenance), smart cities, and video surveillance in the transport sectors all need the underlying 5G infrastructure. One outstanding problem, according to BTS, the branch organization of business telecommunication bulk consumers, is indoor 5G coverage, but it’s only a matter of time until a solution is found.
2nd 5G frequency auction to go ahead
The European Union has decided that three frequency bands will be made available for commercial 5G applications: 700MHz, 3.5GHz, and 26GHz frequencies. The 3.5GHz band has been deemed the most important one to the provision of nationwide 5G in every EU member state.
The use of data is expected to continue apace with the continued rollout of the Internet of Things and 5G. Unlocking, processing, and linking large volumes of data will be easier and faster than ever thanks to developments in artificial intelligence and better algorithms. Based on big data analyses, large amounts of value can be generated. At the same time, there is also a personal aspect to big data. Issues of privacy and ethics continue to rear their heads.
In sum
Of course, this blog couldn’t cover every trend. To wit, we left out some ongoing trends like climate change, trade wars, and refugee streams. Not out of a lack of interest, but because the world is complex enough as it is. Yet there is hope – and not just for the technology evangelists – that these problems may eventually be solvable, or at least controllable, using technologies like AI, BI, and big data analytics.