Plan Do Check Act explanation
PDCA stands for Plan, Do, Check, and Act, a cycle that allows you to start improving your goals, KPIs, and policies in a structural and playful way. In short, this is how it works: make a plan, because a goal without a plan is a vain hope. Execute the plan (Do) and collect as much (process) data as possible and monitor & analyze the information. In the Check step, you check whether the results meet the set goals and target values of the KPIs. Did the plan succeed or fail? Did you meet the standards and targets? Based on that comparison, you adjust the execution or scale up or down (Act). Or you adjust your plan. You don’t go through the PDCA cycle once but as a continuous process: daily, weekly, monthly, quarterly, or annually. Spin the PDCA wheel with regularity and create a flywheel for continuous improvement. View a PDCA example here.
What is PDCA?
To really grasp the PDCA meaning, it is important not only to memorize the PDCA abbreviation but also to understand the essence and live the concept. The order of the four capital letters is often mixed up. So, PDCA stands for: Plan Do Check Act and that is also the only correct order to implement improvement processes. Act does not refer to action, but to actualize.
Figure 1: The steps Plan, Do, Check, Act help you to implement continuous improvement and quality management. But a good plan requires passion, in the do step you use data for analysis, the check step cannot be done without conscious control, and autonomy of action ensures self-direction and reflection in act. More explanation: the 2 variants of PDCA.
What can a PDCA cycle be used for?
With a PDCA cycle, you initiate a continuous learning and improvement process in an organization. You do this by giving KPIs, data analysis and human reflection a leading role. In this way, you give people who are passionate about their work the daily opportunity to improve their work process incrementally, step by step.
What is a PDCA cycle?
A PDCA cycle is a system that ensures that a given value moves around the standard, where you keep raising the bar. The term “cycle” indicates a (self) repeating process. It is also referred to in this context as a loop or control loop, a feedback loop where you measure the output of a process and then compare it to the desired value. Then you start acting on it. For example, reducing waste and defects, shortening the lead time, or improving the quality of the final product.
The basic principles of a PDCA cycle: two variants
How do you build a robust PDCA model? And is there actually a difference between the Deming cycle and the PDCA cycle? The illustration below provides a concise explanation of the four PDCA steps:
- Plan: Define your plans and the expected results | details
- Do: Test the plan and observe what happens | details
- Check: Verify that the results achieved are satisfactory | details
- Act: Implement the method that produced the desired results | details
Figure 2: Overview of the PDCA cycle and the four steps needed to get it working.
The 4 Phases of PDCA
Below we discuss the four phases of PDCA, continuous improvement, and learning processes in organizations. We give the PDCA cycle meaning and more clout by connecting two domains – data analysis and continuous improvement. By adding four new dimensions to Deming’s quality cycle, we give the traditional PDCA cycle a new, modern look. Let your organization also benefit from the latest insights and our unique PDCA tips.
Phase 1 in the PDCA cycle: Plan
In the Plan phase, managers and professionals draw up a plan that includes what results they want to achieve and how they want to do it. In doing so, they take into account any frameworks imposed on the work process as preconditions. Plans and objectives are made SMART with KPIs and standards. This serious planning explicitly includes inspiring your people. The “why” must be crystal clear to everyone.
Phase 2 in the PDCA cycle: Do
In the Do phase, execution takes place, including the recording of events (input, processing, and output). Through interactive dashboards and data analysis, professionals monitor execution. People are allowed to make mistakes, but these are addressed directly at the source. There is room to experiment. In implementation, however, you have to mobilize your people. You will only succeed if you ensure sufficient capacity (so do not accept staff shortages) and deliver quality (well-trained people).
Phase 3 in the PDCA cycle: Check
In the Check phase, executives and professionals compare their actual results achieved with the planned results. They compare the reality with the KPI standard and the plan. To what extent is the execution still in line with the plans and objectives? In practice, there appears to be a large gaping hole between these two worlds: the world of management and that of the shop floor. Intelligent, data-driven organizations see discrepancies as an opportunity to learn. They evaluate the differences (the gap) and figure out their causes (root cause analysis). At this stage, you should also appreciate your people for what they have accomplished.
Phase 4 in the PDCA cycle: Act
In the Act phase, adaptation and adjustment take place. Think of interventions and measures to still achieve the originally planned result. Managers adjust standards and KPIs but keep raising the bar. Employees develop a mindset of getting a little better every day. In this phase, you start reflecting with each other not only on the content but also on the progress of the overall PDCA.
Figure 3: The PDCA cycle cannot exist without an IMAR cycle.
NOTE: Inspiring, Mobilizing, Appreciating, and Reflecting, abbreviated to IMAR, is inextricably linked to these four steps in the traditional PDCA cycle.
Securing without worrying about tomorrow
The PDCA cycle is emphatically not meant to be a one-time exercise. The effort is too intensive and the time commitment too costly. Continuous improvement should always be the goal (see figure 4). Ultimately you want to use continuous improvement cycles to achieve structural quality improvements that will still be acceptable, tenable, and sustainable tomorrow.
Figure 4: Continuous improvement thanks to interim assurance of the results achieved
This means that you will have to secure the often laborious results achieved in order not to have to keep reinventing the wheel. Standardize what works and reject what doesn’t.
PDCA cycle example: make it a sport
As a concrete PDCA example, let’s use a current one for Europeans: reducing your own energy consumption. You want to reduce this because you hate waste, for example. Moreover, it’s also good for your wallet. With energy prices skyrocketing, saving energy is a must.
- Plan: consume a maximum of 150 kWh of electricity and 80 cubic meters of gas monthly. Current consumption is 180 kWh and 125 cubic meters of gas, respectively. Time to act. In your plan, you devise a number of measures to reduce energy consumption, such as turning off the heating one hour earlier in the evening and installing LED lights.
- Do: you implement the measures and every month you receive the consumption data from your energy supplier via an app. You analyze that data and plot it over time. You make a graph of it. You also make the costs transparent.
- Check: the first month after your plan, you discuss your energy consumption with your partner. You are at 179 kWh and 126 cubic meters of gas, severely insufficient to achieve what you planned.
- Act: you add a number of additional measures to your original plan. For example: take a 1-minute shorter shower (applies to all family members) and turn off appliances that use a lot of power, such as a clothes dryer and a television.
Figure 5: PDCA cycle worked out example – energy consumption across months, constantly look for room for improvement.
You go through the PDCA cycle until you reach your goal (the standard). You make it a sport. Or you adjust your goals and standards because your roommates are running cranky through the house due to the shortened shower times. But note that people are creatures of habit. Therefore, even in the above simplified example, it is essential to secure the results achieved, so as not to fall back into old habits (sneak a minute longer shower). At the same time, it is important to look for new savings opportunities. For example, you can also start improvement cycles with the goal of limiting the use of the dishwasher or deactivating the standby mode on all kinds of appliances, for example.
Also in work situations and in almost any business sector, such as healthcare, for example, there are plenty of ambitious improvement projects to define on which you can apply the PDCA principles and achieve continuous improvement. Contact one of our management consultants to discuss and define your project.
The 2 variants of the PDCA cycle
Looking at the positioning of PDCA within a business or work process to achieve improvements, there are two basic forms of PDCA, or two routes to success:
Figure 6: The two basic forms of PDCA visualized
- The light, regular variant: this approach focuses on optimizing existing work processes. You monitor and discuss results using KPIs, make adjustments where necessary, and implement minor improvements – provided the risk of disrupting the process remains acceptable. This variant aligns with the classic planning and control cycle, making use of existing resources, standards, targets, and KPIs. Changes, improvements, or corrections take place within the line organization, without fundamentally altering processes or plans.
- The heavy, experimental variant: this variant is designed for major process changes or innovations. You begin by thoroughly testing solutions to problems – or exploring promising improvements – in a safe “sandbox” environment (the experimentation room) before implementing them in practice. This version of the PDCA cycle starts with fundamental questions about process redesign and often requires new resources, skills, technologies, or ways of working. Only after a successful experiment do you elevate the solution to the standard. At that point, you transition back to the light variant.
Although the two variants differ in scope and intensity, the same KPIs remain applicable.
The SMART KPI Guide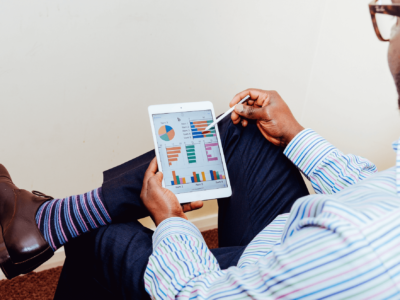
11 PDCA tips and prerequisites for success
Below we provide some tips for implementing the PDCA cycle. Use these tips as prerequisites for the proper application of the PDCA model in your own organization.
- Going through the PDCA cycle pro forma is pointless and a contempt for the Deming circle. Take the example of a healthcare organization where everyone is critically reviewing and monitoring all processes and KPIs ahead of an inspection that will take place in two months. If after the inspection everyone goes back to business as usual, nothing has been achieved. That is precisely not the purpose of the PDCA cycle. PDCA is about continuous improvement and intervening deeply in the organization and should not take place superficially, once a year or so.
- A PDCA without KPIs is like a car without wheels. Don’t forget to name in your plans the desired improvement of one or more KPIs. During the Do phase, you will analyze the data behind the KPIs, in the Check phase you will compare the execution based on your KPIs with your objectives as formulated in the plans or policy intentions. The PDCA circle can never start turning without reliable data, insights, and SMART KPIs. Improve KPIs sustainably based on the rhythm of the PDCA cycle.
- Always apply the PDCA cycle integrally: that is, go through all the steps. People tend to pick only those steps from the PDCA method that are useful to them at that moment. There is cherry-picking. Or they get no further than the P and the D, and give up at the C. So, you never reap all the benefits of the Deming cycle. The integral application of the Deming circle, if properly done with KPIs in the line, will eventually always translate into better (financial) performance.
- Realize that the PDCA cycle can be applied at strategic, tactical, and operational levels. Moreover, the different levels are closely intertwined. The implementation of the strategic plans determines the frameworks for drawing up the tactical and operational plans. The progress reports from the tactical and operational reports from the Check phase serve as input for the higher strategic plans. You will thus begin to see the connection between improvement initiatives, strategic frameworks, and operational processes.
- Regularly test your plans against implementation. Do not wait too long but start with this as soon as possible. There are now enough examples of projects that were started with the best of intentions, but because of a lack of interim adjustment, have gone completely off the rails.
- Make data analysis a permanent part of the Do phase. The PDCA cycle stands or falls with reliable data that you need to analyze in more detail. Without solid data analysis, you’ll never get the PDCA cycle completed and you won’t be able to do your job properly.
- Take the Check phase seriously. For example, set aside half an hour every two weeks for each team and individual employee to complete the Check phase. Is the process running as expected? Does the output meet the standards? Are deviations staying within the rule boundaries? And how is the trend developing? The same motto applies to the Act phase, but then those responsible at the strategic and team levels must get to work.
- Always link the major PDCAs to the minor PDCAs. The decision to only set up a PDCA cycle at the policy level and over a large one-year time frame does not ensure continuous improvement or innovation. A large PDCA cycle alone is too unwieldy for that. And if employees focus solely on their daily improvement in a small PDCA cycle, they lose sight of the larger development. So, both cycles are needed. The large PDCA gives direction and frameworks to the smaller PDCA, allows for in-depth investments, and ensures stability and continuity. The smaller PDCA cycles are improvement loops that are small enough that a person recognizes themselves in them and large enough that a person can measure their own performance against them.
- No longer base organizational decisions on opinions or feelings, but on facts. Inspiring managers will regularly adjust standards and KPIs while raising the bar ever so slightly. Employees, in turn, will develop an improvement mentality. They are “in control” and experience more job satisfaction because their autonomy puts them back in control.
- Make a link between the PDCA and lean processes. Look for symptoms of poor processes such as long lead times, lots of extra checks, lots of data retyping, high work stocks, buffers, and lots of exceptions in the process. Look for the eight wastes that occur almost in every process or organization: overproduction, overprocessing, waiting, transportation, inventories, moves, defects, Deming wheel, and poor use of talent. For each waste, start a new PDCA cycle and create a plan to stop the waste.
- The D of Data and the D of Do: achieve better financial results using PDCA. Our large-scale PDCA research shows that applying Plan Do Check Act company-wide doesn’t improve profit margins only. Work stocks drop dramatically and customers are more satisfied. These results are mainly due to a few simple rules of thumb: set standards and targets for each KPI (Plan), regularly evaluate the standards and targets and adjust them (Act), use steering information and KPIs consistently for analysis and action (Do), use steering information and KPIs to improve and innovate (Do), talk about the numbers and hold each other accountable for negative and positive performance (Check).
If you would like to delve further into the mechanism of the PDCA cycle, download our free article ‘The PDCA cycle is alive and well’.
Article ‘The PDCA cycle is alive and well’
The data-driven PDCA
Adding four new dimensions to the traditional PDCA circle creates what we call a data-driven PDCA cycle. The P of Plan is extended with the P of Passion. The D of Do is connected to the D of Data. The C of Check is associated with the C of Conscious control and the A of Actualize is correlated with the A of Autonomy.
- Passion: every plan ultimately begins with a personal mission, or passion. With someone who really wants something different, who has a dream, or personally feels the urgency or drive to actually improve something.
- Data: just the Do phase is not enough. Doing, based on data analysis, and on irrefutable facts that reliably monitor, over a long period of time, the impact of your attitudes and behaviors, forms the extension of this phase.
- Conscious control: monitoring alone is not all that happens in the Check phase. The constant feedback of data and its effects activates a learning loop. People become increasingly aware of their own role, the role of others and the effect of all these influencing factors on the whole. They learn how the business actually works. And they learn how the (whole) system responds. Conscious control allows people to consciously adjust plans. Thus, they become more and more “in control.”
- Autonomy: the outcome of going through PDCA circles is that the professional develops more and more autonomy. They no longer have to directly follow managers but can determine on the basis of data what works and what needs adjustment.
Following this now amply proven PDCA method, we allow organizations to take maximum advantage of the improvement potential present in every organization. Through this enrichment of the PDCA cycle, we also implicitly respect the ideas of William Edwards Deming. He was a great proponent of the use of statistics and data in the PDCA cycle.
PDCA Cycle = Deming Cycle
In practice, the PDCA cycle, Deming’s cycle, the Deming wheel, and Deming’s quality cycle are used interchangeably. Just to clarify right away: there is no essential difference and all concepts are visualized almost in the same way: as a circle or a wheel. In other words, they are all synonyms for the same concept, the concept that is all about continuous improvement, controlled process optimization, and data-driven work.
Want to know more or need practical advice?
Do you want to know how to improve your organization’s performance with the Plan Do Check Act cycle? Do you want a clear explanation of the PDCA cycle? Are you curious about how you can make your company or teamwork smarter and also more data-driven with the PDCA method? Do you want to know how to draw up a practical PDCA form? Or do you need an improvement consultant or change specialist? Then contact us now for an exploratory meeting with one of our PDCA experts or improvement coaches.
About Passionned Group
Passionned Group is a leading specialist in implementing the PDCA cycle and data-driven PDCA cycles. Our experienced and passionate consultants help larger and smaller organizations in making teams and the entire organization data-driven. Every other year we organize the independent election of the ‘Smartest organization in the Netherlands’.
Frequently Asked Questions
What does PDCA stand for?PDCA stands for Plan, Do, Check, and Act. In this context, Act does not mean action, but actualize.
What is the purpose of a PDCA cycle?
With a PDCA cycle, you initiate a continuous learning and improvement process within an organization, aiming to gradually improve the performance of organizations, teams, and individual employees. Not just once, but continuously.
What are the basic principles of the PDCA cycle?
Establishing standards and targets, using information to improve and innovate, regularly evaluating and adjusting standards and targets, and consistently using information for analysis and action. And last but not least: holding each other accountable for both positive and negative performance.
What is the difference between the PDCA cycle and the Deming cycle?
There is no difference. They are synonyms for the same concept. William Edwards Deming is considered the founder and originator of the original PDCA cycle.
What is the difference between PDCA and Kaizen?
Kaizen is a continuous improvement philosophy focused on making small, incremental changes over time, often driven by everyone in the organization. PDCA is a structured method used to implement and manage those improvements. In short, Kaizen is the mindset, and PDCA is the tool to carry it out.
What is the difference between the PDCA and the data-driven PDCA?
The data-driven PDCA was introduced by Daan van Beek in his PDCA manual “Working Datacratically“. He enriched the PDCA cycle by adding four new elements with identical capital letters: Passion, Data, Conscious Control and Autonomy.
What does the acronym IMAR mean?
IMWR stands for Inspire, Mobilize, Appreciate and Reflect. You always need an IMAR cycle to get the PDCA cycle going.
What are the steps in the quality cycle?
In the Plan phase, you jointly draw up a plan. In the Do phase, implementation takes place. In the Check phase, you compare the actual results achieved with the planned results. In the Act phase, adaptation and adjustment take place.
What is the relationship between the PDCA cycle and lean management?
Within the lean philosophy, eight different forms of waste are distinguished. You can start and run a separate PDCA cycle for each of these wastes, but you can also combine wastes, of course.
Isn’t the PDCA cycle a dated story, a myth?
Absolutely not, the PDCA cycle is alive and well. Using the PDCA, products and services can continuously stay in line with customer expectations. Moreover, an organization’s strategic positioning is more sustainable.
What is necessary while going through the PDCA?
The PDCA cycle is all about support, quality awareness and commitment at all levels. Furthermore, Plan requires passion, Do requires data, Check requires conscious control and Actualize requires autonomy of action.
When is working with PDCA finished?
Never. PDCA’ing means continuous improvement with the emphasis on continuous.