PDCA is never an end in itself
Accelerating and putting your irons in the fire when it’s hot is a hallmark of data-driven working. The cycle doesn’t determine the speed of action, but the urgency to make new plans (Plan), new actions (Do), a new Check, or new adjustments do. It’s not solely about completing the cycle, because that would make PDCA an end unto itself. Instead, it’s about fostering a culture of continuous improvement and responsiveness, ensuring that each iteration brings tangible benefits. By embedding agility into the PDCA cycle, organizations can better navigate the fast-paced and ever-changing business landscape. This dynamic approach empowers teams to swiftly respond to new challenges and seize emerging opportunities with precision and confidence.
Integrate new insights into the greater whole
The Act phase of a small PDCA cycle can reveal that a big PDCA cycle has to be completed faster. Or that a larger PDCA cycle has to be restarted with a new plan. For example, to integrate new insights into the greater whole. The small cycle indicates that the large cycle has to be designed differently.
An earlier PDCA cycle could also lead to the realization that a new Actualize phase would have to involve the suppliers, the citizen, the buyer, the manufacturer, and the inspectors. It shouldn’t be the case that the slower, big PDCA cycle will keep going at its own rate at all times.
As fast as possible and as slow as necessary
The important thing is to arrive at the next, well-founded Act phase as fast as possible but as slowly as necessary. Wherever and whenever the urgency to enrich your original plan (act) may appear. How do you rapidly reach the next Act phase? Some practical examples can clarify this process.
Break free from rigid constraints
Those who think in rigid functions also don’t continue to develop. As the case of the Safety Region North-Holland North demonstrates, it can be very valuable if ambulance personnel starts doing things originally meant to be part of the hospital staff’s function.
Doing things outside of your function or profile increases your agility. Instead of rigid functions like HR specialist, financial employee, sales employee, marketing employee, et cetera, these are becoming more and more different, valuable roles.
This prevents people from identifying too much with a specific function, missing the bigger picture and losing sight of the organization’s overarching goal. That’s a real risk. It helps when people don’t identify 100% with their job description. People can go faster when they are open to delegating some of their tasks to a different part of the process.
The Data Science book for Decision Makers & Data Professionals This brand-new Data Science book covers every essential organizational and technical facet of (big) Data Science, AI, and machine learning. In 10 steps, this Data Science book teaches you how to apply Data Science, algorithms, and machine learning in your organization successfully.
Invest in empowered professionals instead of managers
Many organizations have been working with KPIs, balanced scorecards, and strategy maps for years. Management’s big frustration is that the right information never gets to the right place. Changes take place in the workplace, where professionals work within the primary process. Data-driven working has given us the mechanism to make problems insightful and open for discussion from the bottom up. At the same time, from the top down we can translate changes into policy.
In other words: from the data’s perspective, the executing employee has all the data they need if they have the right dashboard, which eliminates the need for organizations to have lots of managers. This development has empowered and emancipated the people doing the actual work. The professional understands what’s expected of them. The data gives them feedback on what works and what doesn’t, both on a local and individual level as well as on the complete picture.
People used to have to talk about their caseload. Now they can base it on the data, or the system is so data-driven that the caseload will automatically be divided over employees. Employees are thus given more of a voice and are empowered by the data. They can tell their story instead of management giving them a task that’s not sensible, that takes an unreasonable amount of time, and is neither effective nor efficient, and which doesn’t even suit the employee in question.
Go from management-driven to data-driven
At International Bike Group, the power is also shifting from management-driven to data-driven decision-making. Former CEO Davy Louwers says:
Data is very important to us and often leads the decision-making process. If a young business analyst determined that the data revealed the best idea is going left, then we’re going left, no matter what the CEO says.
These developments fit within the growth of Autonomy. Placing authorizations and decisions low in the organization leads to greater agility.
Practical example McDonald’s: 7 lessons learned
Pursuing a dynamic yet profitable product assortment is one of the challenges facing restaurants and retailers. McDonald’s today works with algorithms. Learn from the 7 lessons McDonald’s has already learned.
- Take McDonalds’ dynamic product assortment. That doesn’t only differ per country and per month, but can even differ over the course of a day.
- Products are regularly switched out for other products. Sometimes, burgers that sell well are taken out of rotation, causing managers to think “How can that be? That’s illogical, why is that product being taken out?” It’s not because it’s not selling well or making enough money, but because the data-driven system is saying that other products will become even more popular as a result. In order to enable those other product sales, the system needs the production capacity that’s taken up by the other burger at the moment.
- So if the system decides to switch out a burger that’s selling well, it’s because an algorithm calculated that McDonald’s could optimize the sales of a different burger and sell even more. So the system sometimes makes what appear to be counter-productive decisions that managers wouldn’t make, but that prove to lead to greater revenue and profits after the fact.
- The algorithm’s optimization efforts (artificial intelligence) are constantly being changed. It contains parameters like the season, the weather, day of the week, and the time of day. For example, some McDonald’s products are only sold on certain days. The thought behind it isn’t as simple as “Friday is fish day” – quite the opposite.
- McDonald’s can predict the types of guests on Friday based on past experiences. The company can predict: if we offer this assortment on Friday, we’ll sell products X and Y on time Z. That algorithm is a combination of predictive factors, including historical background and local market forces.
- Playing that game smartly moves the assortment around tactically. Not just based on the question “What are we selling most of?”, but much more dynamically focused on the interplay of questions like “What do we want to sell most of”, “Which guests are we going to have?”, “Which products have which margins?”, “What will demand be like?”, and “Which production capacity do I have to free up and when?”
- That system is more dynamic and volatile than most people realize. Sometimes, certain offers are made to draw a certain crowd. At other times, no sales are offered to keep production capacity for a boost that’s already been predicted before the customers have entered the establishment.
Source: interview with Anouk Schoemaker, former manager at McDonald’s.
In 2019 McDonald’s acquired the startup Dynamic Yield and used its decision technology to further increase personalization and improve customer experience. Another acquisition involves Apprente, which uses voice-based software. Ever since, McDonald’s has been busy with pilots to enable automated drive-thru ordering using artificial intelligence software.
The BI & Analytics Guide™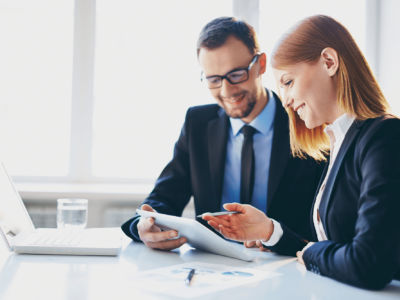
Discover the gap in the market
Besides McDonald’s, there are many other companies where data-driven decisions are increasingly playing a dominant role. Case in point: several years ago, a data-driven marketing agency lined up every brand of car and model, listing all of their properties. They used that to do customer research. That revealed a great demand for a Landrover-esque car, with the driving properties of a city car, a sporty look, and a luxury aesthetic. The marketing agency approached BMW with the idea of making that type of car. Initially, BMW’s chief designer resisted this idea. But eventually, the experiment led to the X5. It proved to be a huge success.
As a result of this data-driven product development, every luxury sports manufacturer – like Audi, Porsche, and Mercedes – started producing successful SUVs. Because the research proved that there was customer demand for it. Factor analysis based on a mathematical model led to the creation of clusters of customers, products, and characteristics, leading to an entirely new market segment.
Conclusion
Empowering professionals and embracing data-driven decision-making using PDCA cycles has led to the creation of entirely new market segments and business models in certain industries.